Publications
2023
Fine-Tuning Deteriorates General Textual Out-of-Distribution Detection by Distorting Task-Agnostic Features (Findings of EACL 2023)
Detecting out-of-distribution (OOD) inputs is crucial for the safe deployment of natural language processing (NLP) models. Though existing methods, especially those based on the statistics in the feature space of fine-tuned pre-trained language models (PLMs), are claimed to be effective, their effectiveness on different types of distribution shifts remains underexplored. In this work, we take the first step to comprehensively evaluate the mainstream textual OOD detection methods for detecting semantic and non-semantic shifts. We find that: (1) no existing method behaves well in both settings; (2) fine-tuning PLMs on in-distribution data benefits detecting semantic shifts but severely deteriorates detecting non-semantic shifts, which can be attributed to the distortion of task-agnostic features. To alleviate the issue, we present a simple yet effective general OOD score named GNOME that integrates the confidence scores derived from the task-agnostic and task-specific representations. Experiments show that GNOME works well in both semantic and non-semantic shift scenarios, and further brings significant improvement on two cross-task benchmarks where both kinds of shifts simultaneously take place.
The paper (Findings of EACL 2023) and code repo are available.
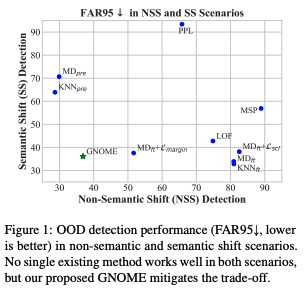
2022
Expose Backdoors on the Way: A Feature-Based Efficient Defense against Textual Backdoor Attacks (Findings of EMNLP 2022)
Natural language processing (NLP) models are known to be vulnerable to backdoor attacks, which poses a newly arisen threat to NLP models. Prior online backdoor defense methods for NLP models only focus on the anomalies at either the input or output level, still suffering from fragility to adaptive attacks and high computational cost. In this work, we take the first step to investigate the unconcealment of textual poisoned samples at the intermediate-feature level and propose a feature-based efficient online defense method. Through extensive experiments on existing attacking methods, we find that the poisoned samples are far away from clean samples in the intermediate feature space of a poisoned NLP model. Motivated by this observation, we devise a distance-based anomaly score (DAN) to distinguish poisoned samples from clean samples at the feature level. Experiments on sentiment analysis and offense detection tasks demonstrate the superiority of DAN, as it substantially surpasses existing online defense methods in terms of defending performance and enjoys lower inference costs. Moreover, we show that DAN is also resistant to adaptive attacks based on feature-level regularization.
The paper (Findings of EMNLP 2022) and code repo are available.
Holistic Sentence Embeddings for Better Out-of-Distribution Detection (Findings of EMNLP 2022)
Detecting out-of-distribution (OOD) instances is significant for the safe deployment of NLP models. Among recent textual OOD detection works based on pretrained language models (PLMs), distance-based methods have shown superior performance. However, they estimate sample distance scores in the last-layer CLS embedding space and thus do not make full use of linguistic information underlying in PLMs. To address the issue, we propose to boost OOD detection by deriving more holistic sentence embeddings. On the basis of the observations that token averaging and layer combination contribute to improving OOD detection, we propose a simple embedding approach named Avg-Avg, which averages all token representations from each intermediate layer as the sentence embedding and significantly surpasses the state-of-the-art on a comprehensive suite of benchmarks by a 9.33% FAR95 margin. Furthermore, our analysis demonstrates that it indeed helps preserve general linguistic knowledge in fine-tuned PLMs and substantially benefits detecting background shifts. The simple yet effective embedding method can be applied to fine-tuned PLMs with negligible extra costs, providing a free gain in OOD detection.
The paper (Findings of EMNLP 2022) and code repo are available.
2021
Translation as Cross-Domain Knowledge: Attention Augmentation for Unsupervised Cross-Domain Segmenting and Labeling Tasks (Findings of EMNLP 2021)
We propose a novel paradigm based on attention augmentation to introduce crucial cross-domain knowledge via a translation system. The proposed paradigm enables the model attention to draw cross-domain knowledge indicated by the implicit word-level cross-lingual alignment between the input and its corresponding translation. Aside from the model requiring crosslingual input, we also establish an off-the-shelf model which eludes the dependency on crosslingual translations. Experiments demonstrate that our proposal significantly advances the state-of-the-art results of cross-domain Chinese segmenting and labeling tasks.
The paper (Findings of EMNLP 2021) and code repo have been released.
2020
Feature Space Singularity for Out-of-Distribution Detection
We propose a simple yet effective algorithm named FSSD based on a novel observation: in a trained neural network, OoD samples with bounded norms well concentrate in the feature space. It achieves state-of-the-art performance on various OoD detection benchmark and enjoys robustness to slight corruption in test data.
The paper (Safe AI workshop at AAAI 2021) and code repo have been released.